The realm of artificial intelligence (AI) is nothing short of mesmerizing. Yet, beneath its dazzling exterior lies an underlying complexity that often keeps AI from realizing its full promise. Indeed, as we marvel at deep learning algorithms that recognize speech or autonomous vehicles that navigate cityscapes, we can’t help but ponder: What’s next? What untapped potential still remains to be explored, and what roadblocks stand in the way? In this incisive article, we delve into the untapped potential of AI, examining both the lofty ambitions we hold for it and the real-world challenges that await. So, buckle up. We’re about to embark on an illuminating journey into the future of AI.
In this article:
- Introduction
- The Dream vs. Reality
- Ethical Considerations
- Technological Hurdles
- Economic Implications
- Case Studies
- The Road Ahead
- Conclusion
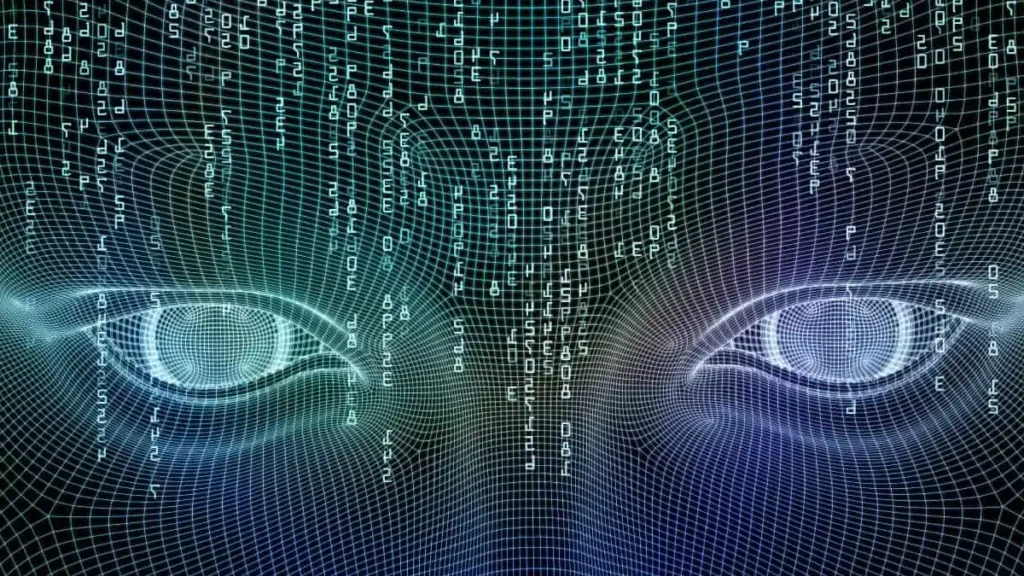
1. Introduction
The dawn of artificial intelligence (AI) ushered in a revolution that rivals the industrial age. With machine learning algorithms detecting diseases earlier than ever, to autonomous vehicles taking the wheel and smart homes creating personalized living experiences, AI’s current successes are nothing short of groundbreaking. Yet, even as we celebrate these strides, we can’t shake the feeling that we’re merely scratching the surface. If data is the new oil, then AI is the refinery, tirelessly working to turn raw information into intelligent action. But like any machinery, it has its limitations. It promises to “live forever” in our societal constructs, continually evolving, adapting, and improving. However, what does it mean for AI to “live forever”? What untapped reservoirs of potential are we yet to discover? What roadblocks are preventing AI from fulfilling its most audacious promises?
In this compelling deep-dive, we will unravel the layers of AI’s unrealized potential. We will confront the ethical dilemmas that plague its expansion, scrutinize the technological bottlenecks that inhibit its capabilities, and survey the economic landscapes that will either propel or hinder its growth. So fasten your seat belts and prepare for a revealing journey through the captivating, yet labyrinthine world of AI’s unrealized potential.
2. The Dream vs. Reality
In the early days of artificial intelligence, the dream was grandiose—machines that could mimic human intelligence in all its glory. We fantasized about robots that would effortlessly understand and respond to human emotions, autonomous systems capable of ethical reasoning, and virtual worlds indistinguishable from our own. Fast forward to today, and what we have are powerful algorithms that excel in specific tasks but fall short of the universal, generalized intelligence we envisioned. In short, the dream isn’t wholly actualized; it’s a work in progress.
The Gap
One might argue that AI’s current accomplishments—such as machine learning models that outperform humans in data analysis, or chatbots that can mimic human conversation—represent a pinnacle of sorts. However, these feats pale in comparison to the aspired dream of AI that not only mimics but understands and improves upon human cognition. This discrepancy between aspiration and reality is glaring, and it beckons us to examine the factors causing this gap.
Technological Constraints
Firstly, let’s talk about computational power. While Moore’s Law has propelled us far, the intricate neural networks envisioned for future AI demand processing capabilities far beyond what’s currently accessible. Even with advances in quantum computing on the horizon, we are years, if not decades, away from the computational power necessary to bring the dream to fruition.
Data Dilemmas
Another critical bottleneck is data. Current AI algorithms require massive, well-annotated datasets for training. The dream of an AI that can learn from a few examples—akin to human learning—is still far from reality. Data acquisition is not only time-consuming but fraught with ethical implications.
Cognitive Complexity
Moreover, the human brain’s complexity remains a mystery, making it an elusive target to emulate. We’re still piecing together how emotions, abstract thought, and creativity manifest neurologically. Without this understanding, building an AI system with human-like cognition remains a distant dream.
Ethical Roadblocks
And let’s not forget the ethical quagmires—be it issues of data privacy, algorithmic bias, or the moral implications of creating machines that might one day surpass human intelligence. These ethical concerns serve as speed bumps on the fast track to realizing AI’s full potential.
In summary, the dream of AI is both tantalizing and elusive. While we’ve made significant strides, the road to fulfilling AI’s ultimate promise is still fraught with challenges—technological, ethical, and conceptual. But, recognizing these roadblocks is the first step in overcoming them, setting the stage for a future where AI might indeed “live forever,” continually redefining its boundaries and exceeding our wildest dreams.
3. Ethical Considerations
As we progress further into the age of artificial intelligence, we grapple with questions that extend beyond the realm of code and algorithms. Ethical considerations, while not new to technology, assume a heightened sense of urgency in the AI space. Why? Because AI, unlike any technology before it, has the potential to replicate, and even surpass, human decision-making capabilities. This immense power brings with it ethical quandaries that must be addressed.
Bias: The Unseen Algorithmic Prejudice
One of the most glaring ethical challenges facing AI today is algorithmic bias. Training an AI model requires data, lots of it. However, if the data is skewed, the model it produces will inevitably perpetuate existing biases. For example, facial recognition technologies that are trained primarily on Caucasian faces tend to misidentify individuals of other ethnicities. This isn’t just an operational hiccup; it’s a serious ethical flaw with real-world consequences that could range from wrongful arrests to healthcare misdiagnosis.
Data Privacy: A Double-Edged Sword
AI thrives on data, but the data it feeds on is often personal, sensitive, and private. The constant collection of user data for training AI models raises significant privacy concerns. Who owns this data? Where is it stored? Who has access to it? Without transparent answers to these questions, the advancement of AI remains stymied by debates on user consent and data security.
How Ethical Concerns Slow Progress
Addressing these ethical issues isn’t just a matter of public relations; it’s a bottleneck in AI’s development. Regulatory bodies, increasingly aware of these concerns, are hesitant to green-light AI deployments without thorough ethical considerations. For instance, European Union’s General Data Protection Regulation (GDPR) has set stringent guidelines on data handling, affecting how AI models are trained and deployed.
Ethical review processes, though necessary, can slow down research and deployment timelines. Moreover, the effort to ‘detoxify’ datasets or reconfigure biased algorithms drains resources, both in terms of time and capital, affecting the speed at which new AI solutions can be brought to market.
In a society increasingly wary of technological overreach, the failure to address ethical issues can lead to public distrust, which in itself is a significant roadblock to AI’s adoption. After all, what good is a revolutionary technology if it can’t earn the public’s trust?
In conclusion, while ethical considerations add complexity to AI’s growth trajectory, they are not to be bypassed or minimized. In many ways, these ethical roadblocks serve as necessary checkpoints, ensuring that as AI aims to “live forever,” it does so in a manner that aligns with our collective moral compass.
4. Technological Hurdles
As we’ve navigated the ethical maze surrounding artificial intelligence, it’s crucial not to overlook another category of constraints—technological limitations. Although AI has come a long way since its inception, breaking through various boundaries, the road to its full potential is still marred by considerable technological hurdles. Let’s unpack some of these bottlenecks.
Computational Power: The Gasoline of AI
AI systems, particularly those based on neural networks, are computational behemoths. These algorithms require significant horsepower to train and run, making computational power a critical factor in AI’s growth. Despite advances in semiconductor technology, we’re beginning to hit the physical limitations of Moore’s Law. The computational requirements for training complex models are exponentially rising, making it harder and costlier to push the envelope further. Thus, while computational limitations may not have felt like a hurdle in the early days of AI, they’re increasingly becoming a barrier to innovation and scalability.
Data Limitations: The Quality and Quantity Dilemma
AI’s insatiable appetite for data is both its strength and its Achilles heel. Not only does it require vast amounts of data, but the quality of that data is equally crucial. Anomalies, missing values, or skewed distributions can severely impair an AI model’s performance. Furthermore, acquiring this high-quality data often involves complex logistical and ethical hurdles, as discussed in the previous chapter. Even if data is abundant, curating it into a form that’s usable for machine learning is a task that’s easier said than done.
The Software Bottleneck: Algorithms and Architectures
While it’s tempting to lay the blame solely on hardware and data, let’s not forget the limitations inherent in the AI algorithms and architectures themselves. Current machine learning models, although robust, are far from perfect. They struggle with tasks that humans find trivial, like common-sense reasoning or understanding sarcasm. Research is underway to develop algorithms that can learn more efficiently, generalize better, and even reason like humans, but we’re still far from achieving these objectives.
Interoperability: The Challenge of Technological Cohesion
The field of AI is vast and fragmented, featuring a wide array of technologies developed for different tasks and industries. The lack of standardization and interoperability across these technologies creates yet another hurdle. Imagine the increase in effectiveness if healthcare AI could seamlessly integrate with transportation AI for emergency services. However, as of now, we’re far from achieving such a cohesive technological landscape.
In summary, the road to AI’s full actualization is fraught with technological roadblocks—ranging from computational constraints to data limitations, and even challenges in software and interoperability. As we look towards a future where AI aspires to “live forever,” it’s essential to acknowledge these challenges, not as insurmountable obstacles, but as problems awaiting solutions. By recognizing these hurdles, we can better strategize on how to leap over them, propelling AI into its next evolutionary phase.
5. Economic Implications
In the discussions around the potential and limitations of artificial intelligence, economic considerations often take a back seat. However, economics plays a pivotal role in determining not only the pace but also the direction of AI development. The economic implications are multifaceted, affecting everything from the cost of AI development to its impact on job markets and social equity.
The High Cost of Innovation
First and foremost, AI development is expensive. Whether it’s the hardware needed for computational power or the human capital involved in research and development, the costs add up quickly. These costs have led to a concentration of AI capabilities within large corporations and elite research institutions. While this has spurred rapid development, it also raises concerns about accessibility and monopolistic control over what could be a transformative public good.
Job Markets: A Double-Edged Sword
The impact of AI on job markets is complex and warrants nuanced discussion. On one hand, AI has the potential to automate mundane, repetitive tasks, freeing human workers to engage in more creative and complex endeavors. On the other hand, the transition is unlikely to be smooth. Jobs in sectors like manufacturing, data entry, and even some aspects of healthcare are already being automated, leading to job displacement. The economy will eventually adjust, but the transitional period could be painful, especially for those without the skills or opportunities to adapt.
Social Equity: The Risk of Widening Gaps
As AI continues to grow, there’s a genuine concern that the benefits may not be evenly distributed. Those with the resources to leverage AI will likely experience disproportionate gains, while marginalized communities may face further setbacks. This inequitable distribution isn’t just a social concern but an economic one. A society where resources and opportunities are concentrated among a select few is less stable and less productive in the long run.
The Ripple Effect
Lastly, AI’s economic implications aren’t confined to the tech industry. From agriculture to healthcare, the technologies are poised to disrupt traditional economic models across the board. This transformation could lead to unprecedented efficiency and innovation but also introduces uncertainties that we must prepare for, both economically and socially.
In conclusion, the economic aspects of AI’s development are like the undercurrents in a river—less visible but immensely powerful. As we strive for a future where AI might “live forever,” integrating seamlessly into our lives, we must consider the economic machinery that fuels its development. Only by understanding these economic implications can we hope to steer AI towards a future that’s not only technologically advanced but also economically sustainable and socially equitable.
6. Case Studies
While theoretical discussions provide invaluable insights, nothing drives home a point like real-world case studies. They allow us to understand the intricacies of artificial intelligence in action—where it shines and where it stumbles. Let’s examine a few compelling instances to gain nuanced perspectives on AI’s capabilities and limitations.
Healthcare: AI in Diagnostic Medicine
Exceeded Expectations: AI algorithms have made significant strides in the field of diagnostic medicine. Tools like Google’s DeepMind have shown proficiency in diagnosing eye diseases, sometimes with an accuracy surpassing human experts. This not only augments medical professionals but also holds the promise of democratizing healthcare, bringing expert-level diagnostics to underserved regions.
Lesson Learned: When carefully designed and rigorously tested, AI can be an invaluable asset in specialized fields like healthcare. However, it also exemplifies the need for domain-specific customization; AI in healthcare doesn’t just involve machine learning but also a deep understanding of medical science.
Autonomous Vehicles: The Road to Self-Driving Cars
Fallen Short of Expectations: Despite early optimism, fully autonomous vehicles are not yet a common sight on our roads. Challenges in perception, decision-making under uncertainty, and safety assurance have slowed down progress. Initial promises of a quick rollout have given way to a more measured approach, riddled with regulatory and technological hurdles.
Lesson Learned: Over-hyping capabilities can lead to public disillusionment. The delay in the rollout of self-driving cars serves as a cautionary tale about the dangers of overselling AI’s readiness to tackle complex, real-world challenges.
Natural Language Processing: Chatbots and Customer Service
Mixed Bag: The application of AI in natural language processing has seen both successes and failures. While technologies like GPT-3 have demonstrated impressive conversational abilities, most customer service chatbots still fall short of providing a seamless, human-like interaction.
Lesson Learned: Progress in AI is often incremental. While we’ve seen incredible advancements in natural language models, the technology is not yet foolproof. This underscores the need for continued investment in research and development, even when partial solutions are already deployable.
Financial Markets: Algorithmic Trading
Exceeded Expectations: AI algorithms are now a staple in the high-frequency trading landscape, executing transactions in milliseconds and scanning multiple markets simultaneously. Their ability to analyze vast datasets and execute trades at speeds no human can match has revolutionized the trading floor.
Lesson Learned: AI’s capability to handle vast amounts of data in real-time can provide a decisive edge in fields that value speed and precision. However, this also raises ethical questions, such as creating an uneven playing field in the financial markets.
In summary, these case studies offer a multi-faceted view of AI, showing us that while we have reasons to be optimistic, caution and due diligence are essential. They provide concrete examples of AI’s capabilities and limitations, adding nuance to our understanding of what it means for AI to “live forever.” By studying these real-world applications, we learn not just what AI can do, but what it should do, informing both our expectations and our ethical considerations as we forge ahead into the future.
7. The Road Ahead
As we step into the future, what are the upcoming technologies and methodologies that hold the promise of pushing AI into its next phase? The aim is to close the gap between dream and reality, to move beyond existing limitations, whether they be ethical, technological, or economic.
Quantum Computing
One of the most promising advancements on the horizon is quantum computing. The potential for vastly improved computational power could break current bottlenecks, enabling more complex and accurate AI models.
Federated Learning
This approach allows for machine learning models to be trained across multiple decentralized devices, holding promise for improved data privacy and utilization. It could alleviate concerns over data monopolies and offer a pathway to more ethical AI practices.
Explainable AI (XAI)
Transparency is often cited as a roadblock to AI’s broader acceptance, especially in critical areas like healthcare and law enforcement. Explainable AI aims to make the decision-making process of AI systems transparent, accountable, and understandable for humans.
Bio-Inspired Algorithms
From swarm intelligence to neural architectures mimicking the human brain, bio-inspired algorithms could offer innovative solutions to existing AI limitations. They hold the promise of making AI more adaptive and better integrated with human activity.
8. Conclusion
We have embarked on an exploration, not just of what AI is capable of today, but of its unrealized potential—of what could be its “oasis” in the coming years. From diagnostic healthcare to self-driving cars, the promise of AI seems boundless. Yet, the path is strewn with ethical dilemmas, technological hurdles, and economic inequalities. These challenges are not insurmountable, but they require concerted effort from technologists, policymakers, and society at large.
The case studies serve as a reality check, offering glimpses into AI’s strengths and shortcomings. But they also point towards the advancements that could propel AI from a useful tool to a transformative force. Innovations like quantum computing and federated learning offer a glimpse into a future where AI could become more than just a buzzword but an integral part of our daily lives.
As we stand on the threshold of this new era, the ultimate question is not just how far AI has come, but how far it could go. Could it “live forever,” ingrained so deeply in our society that its absence would be unimaginable? That dream is not as far off as it might seem, but reaching it will require navigating the complex maze of ethical, technological, and economic factors.
In a world increasingly dependent on technology, the path forward for AI is not just a question for scientists and engineers but for all of us. It’s a collective challenge—one that we’ll need to meet head-on if we want to unlock AI’s full potential.
This concludes our comprehensive look into the untapped potential of AI. The road ahead is long, but it’s a journey worth taking, one that could redefine the very fabric of our society and how we interact with technology. Whether artificial intelligence will “live forever” or remain in the realm of unfulfilled potential is up to us, but one thing is certain: the possibilities are endless, and they’re waiting for us to seize them.