In today’s dynamic tech landscape, Hybrid Cloud is not just a buzzword; it’s a necessity. Offering the best of both private and public cloud environments, Hybrid Cloud has become a cornerstone for businesses seeking flexibility, scalability, and cost-efficiency. However, managing these complex infrastructures is no cakewalk.
Enter Artificial Intelligence (AI). A game-changer in its own right, AI holds the promise of transforming Hybrid Cloud management. Intelligent systems can sift through vast datasets, automate resource allocation, and even enhance security measures. In essence, AI acts as the missing puzzle piece, seamlessly integrating with Hybrid Cloud to optimize its resources.
So, why is AI essential for the future of Hybrid Cloud? Read on to discover how these smart systems are revolutionizing the way we manage, secure, and derive value from hybrid cloud architectures.
In this article:
- The Challenges of Hybrid Cloud Management
- Role of AI in Hybrid Cloud
- Case Study: AI in Hybrid Cloud in Action
- Technologies Powering AI in Hybrid Cloud
- Benefits of AI-Integrated Hybrid Cloud
- Practical Steps for Implementation
- Conclusion
- Further Reading
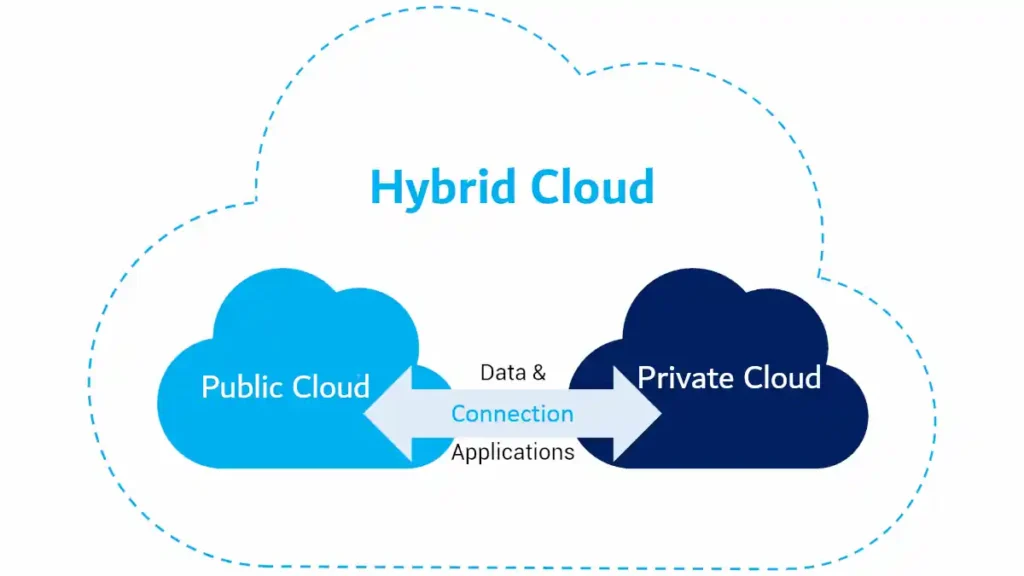
1. The Challenges of Hybrid Cloud Management
As compelling as the advantages of Hybrid Cloud may be, its management isn’t a straightforward affair. From balancing security protocols between different environments to ensuring compliance and cost-efficiency, there’s a myriad of challenges to overcome. In this chapter, we’ll delve into these challenges, dissecting each to understand its complexities.
Security
First and foremost is the matter of security. A Hybrid Cloud environment, by its very nature, is more complex than a single-cloud setup. Data and applications frequently traverse between public and private clouds, making it critical to establish secure pathways and encryption protocols. While the private cloud could be a fortress of security, the public cloud environment might be less so. Therefore, maintaining a consistent security posture across all environments is vital but challenging.
» To read next: Common Security Threats in Computer Networks
Compliance
Next up is compliance. Regulations such as GDPR, HIPAA, or CCPA often require stringent data governance. Managing compliance becomes intricate when data resides in multiple geographical locations and different types of cloud environments. With varying compliance standards across regions and cloud providers, ensuring universal compliance is a Herculean task.
Resource Management
Resource management, too, can be a maze of complexity. It’s not just about allocating CPU, memory, or storage; it’s about optimizing these resources for performance, availability, and cost. Dynamic scaling is a great feature, but it can lead to resource sprawl if not carefully managed. Misallocated resources not only eat into budgets but can also cause performance bottlenecks, affecting user experience and operational efficiency.
Cost-Effectiveness
Lastly, there’s the issue of cost-effectiveness. On paper, Hybrid Cloud seems like a cost-efficient solution, offering the flexibility to scale up or down as needed. However, this flexibility can be a double-edged sword. Without meticulous planning and monitoring, costs can quickly spiral out of control. Think about data transfer fees, API call charges, and the overhead costs of managing a dual-environment system. These ‘hidden’ expenses can add up, negating any cost benefits initially perceived.
In summary, while Hybrid Cloud offers unprecedented flexibility and scalability, it brings along a unique set of challenges in security, compliance, resource management, and cost-effectiveness. Addressing these challenges requires not just technical prowess but strategic foresight. And this is where Artificial Intelligence enters the fray, offering solutions that are both innovative and practical. But more on that in the next chapter.
Navigating these challenges is anything but simple; however, they are not insurmountable. As we transition into a future where Hybrid Cloud is the norm, intelligent systems are not just beneficial but indispensable in mastering these challenges. Stay tuned.
2. Role of AI in Hybrid Cloud
As we’ve discussed, managing a Hybrid Cloud environment is fraught with challenges. From the labyrinthine intricacies of security and compliance to the ever-evolving maze of resource management and cost-efficiency, the difficulties are numerous and complex. However, Artificial Intelligence (AI) emerges as a beacon of hope in this landscape, offering innovative solutions to these challenges. In this chapter, we’ll explore how AI fundamentally alters the equation, providing smarter approaches to resource allocation, predictive maintenance, and security.
Automated Resource Allocation
First on the docket is automated resource allocation. Remember the resource sprawl we mentioned earlier? AI has the capability to mitigate that. Utilizing machine learning algorithms, intelligent systems can continuously monitor workload requirements and performance metrics. These systems dynamically allocate or deallocate resources such as CPU, memory, and storage in real-time, ensuring optimal performance without unnecessary costs. It’s a win-win—resources are efficiently used, and expenditures are kept in check.
» To read next: What is the Turing Test?
Predictive Maintenance
Next, we move on to predictive maintenance—a domain where AI truly shines. Traditional cloud maintenance often involves reactive measures, where problems are addressed after they manifest. This approach, while effective to some extent, is far from ideal. AI changes this by enabling proactive maintenance. Machine learning models can analyze historical data to predict potential failures or downtimes. This foresight allows for preventive measures to be taken before a problem escalates, thereby ensuring uninterrupted service and greater reliability.
Anomaly Detection for Enhanced Security
Last but not least is the role of AI in security, specifically in anomaly detection. Traditional security measures often rely on predefined rules or signatures to identify threats, a method that is increasingly becoming obsolete in the face of sophisticated cyber-attacks. AI, with its ability to analyze patterns and learn from them, offers a more dynamic solution. It continuously monitors network traffic and system behavior, flagging any abnormal activity in real-time. Such quick detection enables immediate action, thereby minimizing potential damage and enhancing overall security.
To sum it up, AI plays an indispensable role in overcoming the inherent challenges of Hybrid Cloud management. It not only automates tedious tasks but adds a layer of intelligence that is critical in today’s fast-paced, ever-changing technological landscape. By integrating AI into Hybrid Cloud systems, we are not just streamlining operations but revolutionizing the very way we perceive and manage cloud computing.
In the next chapter, we’ll delve into a real-world example that encapsulates the synergy between AI and Hybrid Cloud, demonstrating how this blend can achieve unprecedented operational excellence. Stay tuned!
3. Case Study: AI in Hybrid Cloud in Action
Up until now, we’ve discussed the theoretical advantages of integrating AI into Hybrid Cloud management. But theory is just the starting point; the true test lies in practical application. In this chapter, we’ll dive into a hypothetical case study that brings these concepts to life, showcasing how AI dramatically improves efficiency, security, and cost management in a Hybrid Cloud setting.
The Scenario: Globex Corp
Let’s imagine a multinational corporation—Globex Corp—that relies heavily on Hybrid Cloud infrastructure. They have confidential data that needs the stringent security of a private cloud, along with customer-facing apps that benefit from the scalability of a public cloud. Their challenges are typical: maintaining robust security protocols, ensuring compliance across borders, effective resource management, and, of course, cost-efficiency.
The Challenge
Over time, Globex Corp starts facing recurring issues—security vulnerabilities, resource allocation inefficiencies, and escalating costs. The final straw comes when a critical application experiences downtime due to a resource bottleneck, impacting customer trust and satisfaction. The company realizes that their current approach to Hybrid Cloud management is unsustainable and looks for an intelligent solution.
The AI-Enabled Solution
To counter these challenges, Globex Corp integrates AI into its Hybrid Cloud management system. Let’s explore how each challenge is addressed:
Automated Resource Allocation:
A machine-learning model is trained to analyze workload patterns, resource utilization, and performance metrics. The model begins to allocate resources in real-time, taking into account the criticality of the application, upcoming traffic surges, or lulls. The result? No more resource bottlenecks, and the utilization rates are optimized, saving costs.
Predictive Maintenance:
AI algorithms start monitoring system health, identifying potential issues before they become critical problems. For instance, it notices a disk nearing its end-of-life stage and preemptively migrates the data, thereby avoiding a catastrophic failure.
Anomaly Detection for Enhanced Security:
Machine learning models analyze traffic patterns and system accesses. They quickly identify abnormal behaviors that don’t fit typical user patterns. A potential security breach is flagged and isolated long before traditional security measures would have caught it, thereby averting a potential data disaster.
The Outcome
The results are transformative. Not only does the AI integration lead to a more efficient and stable system, but it also translates into tangible financial gains. Operational costs are lowered by 20%, and customer satisfaction rates soar due to the increased uptime and robust security measures.
Moreover, the compliance team has an easier job ensuring data governance. AI models help identify which data sets fall under specific regulatory restrictions, making it easier to maintain compliance without hindering functionality.
» To read next: HTTP Header Vulnerabilities
Conclusion
Globex Corp’s story illuminates the significant benefits of integrating AI into Hybrid Cloud management. They moved from a reactive stance, fraught with inefficiencies and vulnerabilities, to a proactive, streamlined operation. This example demonstrates that the marriage between AI and Hybrid Cloud is not just beneficial but essential for organizations looking to leverage the best of both worlds.
And this is just the beginning. The field of AI in Hybrid Cloud is ripe for innovation, promising even more sophisticated solutions in the near future. Stay tuned for our next chapters as we explore these exciting advancements further.
4. Technologies Powering AI in Hybrid Cloud
The magic of AI in Hybrid Cloud management doesn’t happen in a vacuum. It’s powered by an array of advanced technologies that work in unison to bring intelligence and automation to your cloud infrastructure. In this chapter, we’ll take a swift tour of the technologies that lay the foundation for AI in Hybrid Cloud, from machine learning models to neural networks and beyond.
Machine Learning Models
The backbone of AI’s application in Hybrid Cloud is undeniably machine learning. Various types of models—including supervised, unsupervised, and reinforcement learning—are employed to make sense of complex data sets. For example, supervised learning models are often used in predictive maintenance, training on historical data to foresee future system failures.
» To read next: When I Grow Up I Want To Be a Data Scientist!
Neural Networks
Neural networks, particularly deep learning architectures, offer another layer of sophistication. These networks mimic the human brain’s ability to learn from data, making them particularly effective for tasks like image recognition or natural language processing. In Hybrid Cloud settings, they can be utilized for advanced security protocols like identifying malicious activities based on intricate patterns.
» To read next: Do You Know What Perceptrons Are?
Containers and Microservices
The modularity provided by containers and microservices plays a critical role in the effective deployment of AI in a Hybrid Cloud environment. These technologies allow machine learning models to be isolated and scaled independently, which is essential for handling diverse workloads efficiently.
APIs and SDKs
The interaction between AI algorithms and cloud resources is often facilitated through APIs (Application Programming Interfaces) and SDKs (Software Development Kits). These tools offer a seamless way to integrate machine learning capabilities into existing cloud management systems without the need for extensive code overhauls.
Data Lakes and Warehouses
Data is the fuel that powers AI, and Hybrid Clouds often incorporate data lakes or warehouses to store this valuable resource. Advanced analytics tools can sift through this data, ensuring that the machine learning models are fed high-quality, relevant data for training and inference.
Edge Computing
Last but not least, edge computing is becoming increasingly crucial in Hybrid Cloud environments equipped with AI. Edge computing allows for data processing to occur closer to where it is generated, which is essential for real-time analytics and low-latency responses—key attributes for successful AI applications.
Conclusion
While this chapter offers just a snapshot, the technologies mentioned are integral for the successful implementation of AI in Hybrid Cloud systems. Each plays a specific role, and when used in tandem, they form a robust framework that transforms cloud management from a cumbersome task into a streamlined, intelligent operation.
For more detailed insights into each of these technologies, keep an eye out for our upcoming specialized articles. The future of AI in Hybrid Cloud is not just promising; it’s already here, changing the landscape in profound ways. Stay tuned as we continue to explore this riveting subject in future chapters.
5. Benefits of AI-Integrated Hybrid Cloud
The integration of AI technologies into Hybrid Cloud environments isn’t just a neat technological feat; it’s a practical, high-impact solution that addresses some of the most pressing challenges in cloud management today. In this chapter, we’ll focus on the manifold benefits that come with combining the computational prowess of AI with the flexibility of a Hybrid Cloud system.
Cost-Efficiency
One of the most tangible benefits of AI integration is the significant reduction in operational costs. Automated resource allocation models can optimize the use of cloud resources, eliminating wasteful over-provisioning. Predictive analytics can also identify potential issues before they escalate, reducing the financial burden of emergency interventions and system downtime. Simply put, AI can make your Hybrid Cloud run like a well-oiled machine, maximizing ROI and minimizing unnecessary expenditures.
Scalability
Scalability is often cited as one of the primary benefits of cloud computing. Still, AI takes it to another level by automating and optimizing the scaling process in real-time. Machine learning algorithms can analyze workload patterns, automatically scaling resources up or down as demand fluctuates. This dynamic scaling not only improves user experience but also contributes to cost-efficiency, as you pay only for the resources you actually use.
» To read next: Problem-Solving in AI
Enhanced Security
Security remains a perennial concern in cloud environments, especially those that mix public and private resources. AI technologies, particularly machine learning models and neural networks, can automatically monitor and analyze massive data streams for signs of cyber threats. They can identify anomalies or suspicious activities, taking swift action before human operators might even notice there’s an issue. This proactive security stance can be a game-changer in defending against ever-evolving cyber threats.
Reliability
A well-managed Hybrid Cloud is a reliable Hybrid Cloud. AI algorithms can predict and preemptively address issues that would typically cause service interruptions or downtimes. For instance, machine learning algorithms can forecast hardware failures or identify inefficiencies in load distribution, enabling proactive measures that improve service reliability. As a result, businesses can offer more consistent service quality, thereby increasing customer satisfaction and loyalty.
Conclusion
From cost-efficiency and scalability to enhanced security and reliability, the benefits of integrating AI into Hybrid Cloud systems are manifold. These advantages aren’t just theoretical; they have a direct and measurable impact on an organization’s bottom line and operational excellence. By intelligently leveraging these technologies, companies can not only survive in today’s competitive landscape but also thrive, setting new standards for what is achievable in cloud management.
As we move forward into an increasingly data-driven future, the synergy between AI and Hybrid Cloud will only continue to grow, unlocking new possibilities and redefining what we consider “state of the art” in cloud computing. Stay tuned for our final chapter, where we will explore future trends and the road ahead in AI-integrated Hybrid Cloud systems.
»To read next: The kNN Algorithm
6. Practical Steps for Implementation
Embarking on the journey of integrating AI into your Hybrid Cloud environment is a monumental step, fraught with challenges but also ripe with opportunities. This chapter aims to provide you with a concrete, step-by-step guide to make this integration as smooth as possible. Let’s delve into the practical steps that can turn your AI-empowered Hybrid Cloud aspirations into reality.
Step 1: Assess Current Infrastructure and Goals
Before anything else, take stock of your existing Hybrid Cloud setup and organizational goals. This involves auditing your current hardware, software, and data storage solutions, as well as defining what you aim to achieve with AI integration—be it cost savings, enhanced security, or scalability.
Step 2: Identify Skill Gaps
AI implementation often requires specialized skills. This is the stage to identify any skill gaps in your team concerning machine learning, data analytics, and cloud management. If necessary, invest in training or consider hiring experts.
Step 3: Choose the Right Vendors
Not all cloud providers or AI solutions are created equal. Conduct a thorough vetting process to choose vendors that not only meet your technical specifications but also align with your company’s values, budget, and long-term strategy.
Step 4: Develop a Data Strategy
Good AI needs good data. Formulate a data strategy that defines what data will be collected, how it will be stored, and how you intend to maintain data quality and security.
Step 5: Build or Choose Machine Learning Models
Depending on your specific needs and in-house capabilities, you may opt to build your own machine learning models or leverage pre-built solutions. Either way, ensure that the models you choose are compatible with your overall Hybrid Cloud architecture.
Step 6: Pilot Testing
Before full-scale implementation, run a pilot test to validate your chosen models and architecture. Use this phase to identify bottlenecks, optimize resource allocation, and improve your implementation strategy.
Step 7: Full-Scale Implementation and Monitoring
Once the pilot is successful, proceed to full-scale implementation. However, your work isn’t done yet. Ongoing monitoring is crucial to ensure that the system is delivering the desired benefits and to catch any issues before they become critical.
Step 8: Train Your Team
Ensure that your team is adequately trained to manage the new AI-integrated Hybrid Cloud system. This may involve formal training programs, workshops, or on-the-job training.
Step 9: Iterate and Optimize
The world of AI and cloud computing is constantly evolving. Make it a routine to revisit your setup, update your models, and optimize your architecture to adapt to new challenges and opportunities.
Step 10: Measure and Report
Finally, it’s crucial to measure the impact of AI on your Hybrid Cloud system. Use key performance indicators (KPIs) aligned with your initial goals to gauge success and report these findings to key stakeholders.
Conclusion
Integrating AI into your Hybrid Cloud doesn’t have to be a leap into the unknown. By following this structured approach, you can mitigate risks and maximize the immense benefits that come with AI-empowered cloud management.
So there you have it—a comprehensive guide to usher you into the future of Hybrid Cloud systems. The integration of AI technologies is not just a trend but a revolutionary shift that is redefining what we can achieve with cloud computing. Take the first step today, and you’ll be well on your way to a smarter, more efficient, and more secure cloud ecosystem.
7. Conclusion
As we’ve navigated through the intricate landscape of AI-integrated Hybrid Cloud systems, several key takeaways have emerged. The synergy between AI and Hybrid Cloud is not only revolutionary but also immensely practical, addressing real-world challenges from cost-efficiency and scalability to security and reliability. The future of Hybrid Cloud is unmistakably intertwined with advancements in AI, as machine learning models and other intelligent systems become increasingly crucial for optimizing cloud resources.
If there’s one thing to take away from this guide, it’s that the integration of AI into your Hybrid Cloud environment is not a fleeting trend but a fundamental shift. Companies that successfully integrate these technologies will not only gain a competitive edge but will also set new benchmarks in operational excellence, innovation, and customer satisfaction.
As we look toward the future, it’s clear that the boundary between AI and Hybrid Cloud will continue to blur, bringing forth new possibilities, solutions, and even challenges that we can’t yet fully comprehend. To not only adapt but to thrive in this dynamic landscape, it’s crucial to begin your journey into AI-integrated Hybrid Cloud today.
» To read next: What are Finite State Machines?
8. Further Reading
For those eager to dive deeper into this fascinating subject, here are some additional resources that can provide more in-depth knowledge and practical insights:
Books:
- “Architecting the Cloud: Design Decisions for Cloud Computing Service Models” by Michael J. Kavis
- “Machine Learning Yearning” by Andrew Ng
- “The Cloud Adoption Playbook: Proven Strategies for Transforming Your Organization with the Cloud” by Moe Abdula, Ingo Averdunk, Roland Barcia, Kyle Brown, and Ndu Emuchay
Tutorials:
- AWS Machine Learning Services
- Azure AI and Machine Learning
- Google Cloud AI and Machine Learning Products
Online Courses:
- Coursera: “Machine Learning for Business Professionals”
- Udemy: “Hybrid Cloud Management with Azure & AWS”
Academic Journals:
- Journal of Cloud Computing: Advances, Systems and Applications
- Journal of Artificial Intelligence Research
Websites & Blogs:
Whether you are a seasoned cloud architect, a machine learning enthusiast, or simply someone intrigued by the unlimited possibilities that the fusion of AI and Hybrid Cloud offers, these resources are excellent starting points. Keep learning, keep experimenting, and most importantly, keep pushing the boundaries of what you think is possible.